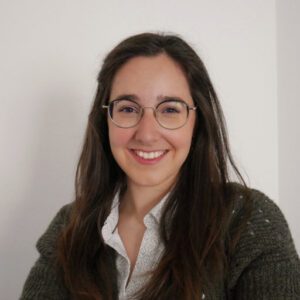
Uncertainty quantification in reactive micrometric processes: toward reliability concerns of CO2 mineral storage at the pore scale.
Sarah Perez (Univ PAU)
Room E007
Abstract
Investigating reactive flows in pore-scale porous media requires estimating mineral reactivities and kinetic parameters, often plagued by discrepancies that introduce modeling uncertainties. Pore-scale modeling of reactive flows also relies on X-ray microCT experiments, prone to image artefacts such as noise and unresolved morphological features, adding imaging uncertainties to the workflow.
To enhance the reliability of direct numerical modeling in micrometric reactive processes associated with CO2 mineral storage, we address the challenges arising from these two types of uncertainties. A deterministic approach is first proposed based on upscaling the microCT unresolved features to estimate macro-permeability uncertainty ranges.
We then present a stochastic deep learning methodology based on a Bayesian Physics-Informed Neural Networks (BPINNs) formulation of multi-objective inverse problems, incorporating the PDE constraints and data fidelity as tasks. We establish a new strategy of automatic task balancing and introduce a robust adaptive weighting of the target distribution in this Bayesian context by leveraging gradient information of the different objectives.
After demonstrating the efficiency of this method, we showcase its applications in reactive inverse problems at the pore-scale, combining imaging and modeling uncertainties to capture both unresolved morphological features and reliability ranges on the reactive parameters.